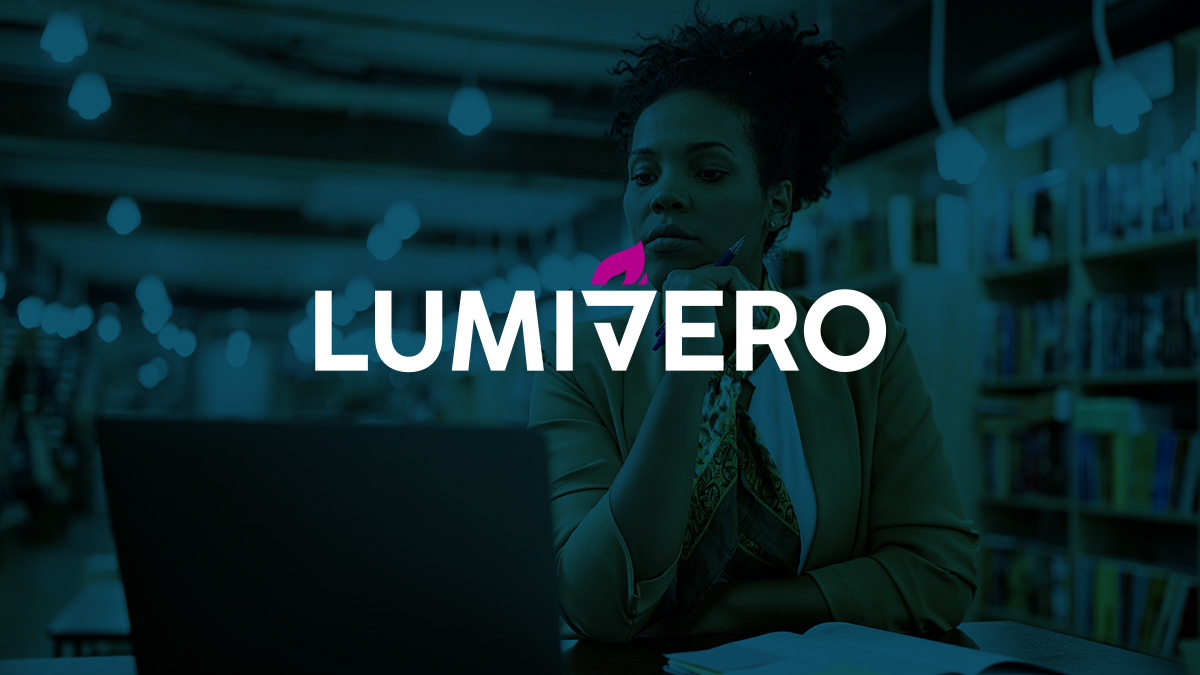
When using @RISK (risk analysis software for conducting Monte Carlo simulations in Microsoft Excel), one of the output graphs is a tornado graph. Such graphs have their most direct interpretation for linear models with independent input distributions, such as in most typical cost budgeting models. In these cases, the regression coefficients provide a measure of how much the output would change if the input were changed by one standard deviation (the correlation coefficients provide a broadly similar measure, but are slightly different as is covered in another posting).
For models which have dependencies between the input distributions (e.g. correlation or parameter dependencies) and models where the output behaves in a non-linear way with some of the inputs, these statements hold either with some qualification or may not hold at all. In such cases, the interpretation of the coefficients will in general be specific to the nature of the model. For example, in linear models with correlated input distributions, the regression coefficient will still provide a measure of how much the output would change if the input were changed by one standard deviation, but only assuming that such a change could be implemented independently i.e. without affecting the other variables.