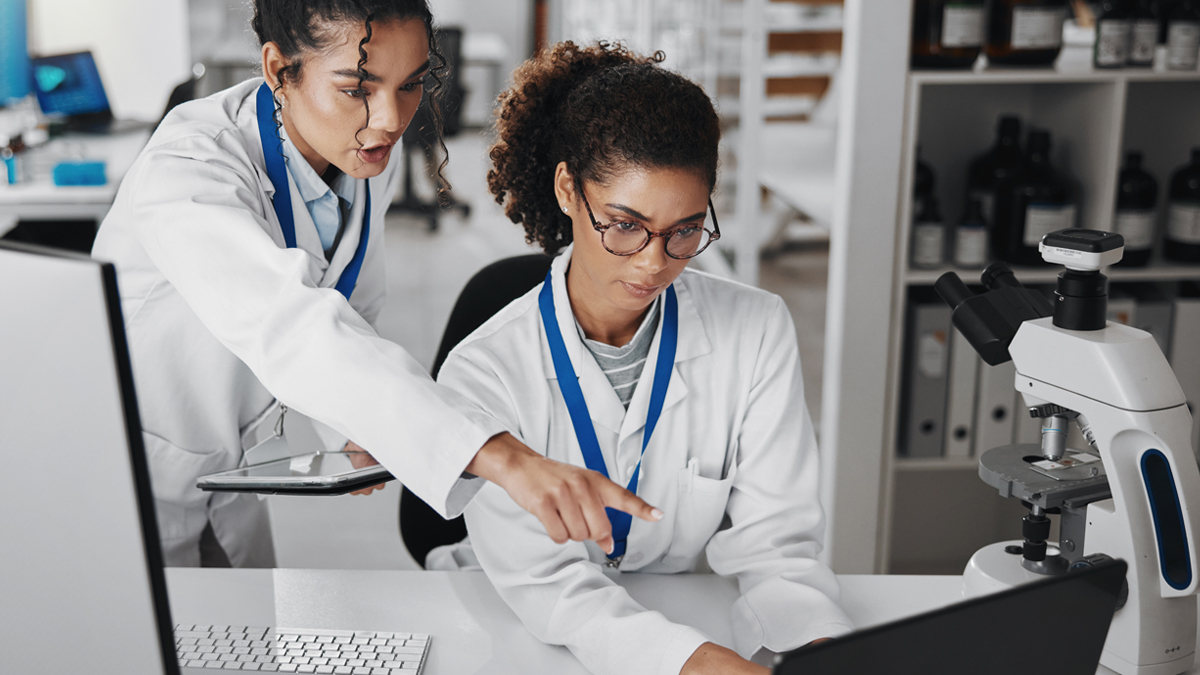
It’s well known that qualitative data analysis software (QDA software) provides significant advantages to researchers, particularly regarding time saved on clerical work which “frees the researcher to engage in the more meaningful work of analysis." Using it is one of the most critical steps in engaging in practical and effective research. However, it is one thing to know you need an analysis tool and another to understand why and when to apply QDA software in your research.
This research analysis guide to qualitative data analysis techniques will help you uncover some of the best practices to ensure the integrity of any analysis you make. Discover practical applications for different methods that can boost your work's accuracy and help you start analyzing qualitative data with greater insight.
Introduction to Qualitative Data Analysis
Due to the complex, non-numerical value nature of qualitative data, qualitative data can be more complicated to analyze than quantitative data. This type of analysis can include data in a wide variety of forms including:
- Interviews
- Focus Groups
- Observations
- Texts or documents
- Imagery
- Audio/video recordings
- Social media data
Due to this, qualitative research tends to:
- Explore subjective experiences
- Explore real-world environments to get authentic experiences
- Allow a flexible, in-depth exploration of subjects
Qualitative data analysis is vital as it can often help uncover insights that quantitative data might miss or offer context to the data that might otherwise go unnoticed – leading to a deeper understanding of the research topic.
At the same time, such research can often demand specialized skills and tools to help analyze the result effectively. Qualitative research often results in large, complex datasets. The more extensive the data, the longer it takes to analyze – resulting in the essential need for QDA software.
Understanding the Importance of Analyzing Qualitative Data
Qualitative data analysis provides a deeper understanding of the data as it digs into the underlying feelings and motivations. This method is commonly used in the following industries:
- Healthcare
- Education
- Marketing
- Psychology
- Political science
- Anthropology
- Sociology
Information gleaned from qualitative data analysis can provide vital insight into behaviors, motivations, perceptions and more – all which are difficult to quantify.
However, due to its complexity, qualitative data requires careful interpretation to find meaningful patterns and demands a high level of transparency and rigor. Using data analysis software, such as NVivo, can help to facilitate a more efficient and in-depth analysis than might otherwise be possible.
Popular Qualitative Data Analysis Techniques
Many qualitative research methods exist, each offering various advantages depending on the research questions of the study. Each technique serves different purposes and can contextualize the same data differently. As such, it is essential to understand their nuances so that you choose the right method for your research questions, design and data type.
Thematic Analysis
Thematic analysis can be used to help you understand the core meaning of your dataset by working to identify and analyze patterns or themes within qualitative data.
This method involves grouping, or "coding," different sections of your collected data and creating thematic maps to help you explore the relationships between them. You can then discuss or write about each theme, deepening your understanding their relationship.
While useful on its own, this form of analysis is very adaptable. You may benefit from using thematic analysis when examining things such as:
- Experiences
- Motivations
- Perceptions
- Attitudes
- User Feedback
Thematic analysis accommodates a wide variety of data types (e.g., documents, interviews, social media posts, images, videos) and is not a rigid methodological framework – allowing for adaptation and flexibility in your research. This method is quite straightforward, making it accessible to researchers at any stage in their career.
Thematic Analysis Example: A study on organizational challenges and adaptations in remote work settings.
- Focus: Experiences of remote workers across a diverse range of industries. Are certain types of jobs more conducive to remote working? Are certain types of employees more comfortable with remote working?
- Data collection: In-depth interviews with a range of remote workers.
- Analysis 1: Start with reading through data to get familiar with it. Start generating initial codes, such as ‘technology adaptation’, ‘communication barriers’, and ‘work-life balance’.
- Analysis 2: Collate initial codes into potential themes. For example, ‘technological adaptation’ and ‘communication barriers’ could form a broader theme of ‘technological challenges’. Then review these themes and refine them until you come to final themes and can relate them to the research questions.
- Report: The report involves weaving the narrative with vivid extracts showing how they support the themes. It might highlight, for example, how technological challenges have spurred innovative solutions.
Watch On-Demand: Introduction to Thematic Analysis >>
Content Analysis
Content analysis is a structured method of data interpretation that interprets data based on the frequency and context of words or symbols it contains. While this is useful for uncovering underlying patterns or trends in qualitative data, it can be considered a quantitative method of data analysis.
Content Analysis Example: A study on social media's role in shaping public opinion on climate change.
- Focus: How does social media discourse influence public perceptions and opinions on climate change?
- Data collection: Data is gathered from several social media platforms (e.g., X (Twitter), Facebook, Reddit. Posts, comments, discussions that mention climate change, global warming etc.)
- Analysis 1: The researcher identifies recurring words/phrases such as ‘carbon footprint’, ‘sustainable practices’, ‘protest’, and look at their frequency. The words/phrases are coded.
- Analysis 2: The coded data are organized to reflect broader themes such as ‘environmental impact’, ‘activism’, ‘denial’ etc. The researcher then interprets how each theme shapes public opinions, how often each theme appears, sentiment of discussion, and who are the influencers.
- Report: The findings are put in a report that discusses the prevalence and impact of each theme.
Read Navigating Inductive Content Analysis in Qualitative Research >>
Many qualitative research methods exist, each offering various advantages depending on the research questions of the study. Each technique serves different purposes and can contextualize the same data differently. As such, it is essential to understand their nuances so that you choose the right method for your research questions, design and data type.
Grounded Theory
Grounded theory is another common method that, while feeling similar to thematic analysis, differs in that it focuses on using data to build the theory. This is an inductive approach that systematically uses the collected data to create a new theory – going further than the surface level of the data. In addition, data collection and analysis is iterative, with initial analysis of the first few data collected, direct where or who should be interviewed next.
The discovered concepts are then used to generate theories during data analysis. Processing the concepts this way encourages the development of more profound "core theories" that can serve as the foundation. As new data comes in, it can be compared with existing codes and categories due to its highly iterative nature.
The social sciences often use this method to understand more complex social interactions, but it demands a flexible approach to interpreting data.
Grounded Theory Example: A study on the coping strategies of students experiencing academic stress.
- Focus - An open topic: No preconceived theories
- Data Collection/ Analysis: In-depth interviews with a diverse range of students; interviews are analyzed as they are being collected to direct the next set of interviews. Data collection stops when no new insights are discovered (saturation).
- Initial coding: ‘Open coding’ derived from participants’ language (e.g., study group, exercise, seeking help, procrastination) – when an expression from a respondent is used as a code, it is called an in-vivo code (e.g., ‘hitting the books’, ‘caffeine fix’).
- Developing categories: Initial codes are grouped into categories such as study group and seeking help can be grouped under ‘social support’ while exercise can be categorized under ‘physical health’.
- Finding the core category: From going through the data ‘social support’ seems to be the key category that connects many aspects of how they cope with stress. It seems central to the theory being developed that influences emotional well-being and academic performance.
- Building a theory: A theory emerges from the interconnection of categories. It might propose that “social support networks are central to effective stress management among college students”.
- Verification: The theory is tested by interviewing new participants – perhaps in different contexts (e.g., exam prep stress vs. coursework deadlines). Verification is done to see if the theory holds or needs adjustments.
Watch On-Demand: An Introduction to Constructivist Grounded Theory >>
Narrative Analysis
When trying to understand personal accounts, especially those in stories, narrative analysis can help make sense of the research participants' experiences. It analyzes several parts of the gathered data such as:
- Structure
- Context
- Content
- Themes
From there, you can then explore the text to discover how people interpret their own experiences and how individuals construct stories. The information gathered can offer context to the rest of the data collected.
When trying to understand various social phenomena or contextualize a specific piece of data, narrative analysis offers distinct advantages due to its ability to:
- Capture complex experiences
- Highlight areas where subjectivity is impacting data
- Reveal undiscovered meanings
- Explore how people construct and adapt their identities
- Help explain social structures and interactions
Narrative Analysis Example: A study on understanding the impact of military service on family dynamics.
- Focus: How does the veteran express their feelings, talk about the changes in relations and their coping mechanisms. Each story will be unique, but you need to understand the context.
- Data collection: In-depth interviews with veterans returning after active military service about their experiences before, during, and after deployment on how it impacted their family relationships.
- Analysis: Looking at common themes such as ‘transition challenges’, ‘communication gaps’ etc. Also look at the structure of their narratives, how they frame their reunion with their families, and the evolution of their identity from a soldier to a civilian and family member.
- Report: The report could be a series of case studies, highlighting the personal and emotional experiences with insights on broader patterns and issues faced by military families. It could inform policymaking on military family welfare.
Watch On-Demand: Narrative Portraits in Qualitative Research >>
Phenomenological Analysis
Put most simply, phenomenology studies an individual’s lived experience in the world. More specifically, it is the study of phenomena as someone experiences it and their perception and understanding of the phenomena. Phenomenological analysis can uncover the meaning behind reported phenomena in a person’s daily life.
According to Neubauer, B. E., Catherine T. Witkop, C. T., Varpio, L, (2019), one of the main challenges in this process is that the researcher must suspend their own attitudes, beliefs, and suppositions to allow focus on the participants’ experience of the phenomenon.
The phenomenological analysis method retains a participant's subjective perspective and often their unique insights based on their background. In this way, it offers a richer, more in-depth understanding of the experience in question.
Phenomenology Example: A study on the experience of attending a music festival.
- Focus/ Research Question: What it feels like emotionally and sensory-wise to be part of a music festival.
- Data collection: In-depth conversations with attendees – what they felt, saw, heard and thought throughout the festival.
- The goal: To understand the ‘essence’ of what it feels like to be part of a music festival. Looking for common themes – excitement, discomfort, camaraderie etc.
- Report: Describing as close as possible what it truly felt to be at the festival based on their accounts. The aim is to make someone who has never attended a music festival feel as if they are there, experiencing it themselves.
Read Post-Intentional Phenomenology: Considerations and Principles >>
Case Study Method
A case study method will examine a specific thing in the world in great detail, often attempting to answer questions of “how” and “why”. The subject of this analysis may be:
- An individual
- An organization
- An event
- A process
- A place
- An object
This method allows for an in-depth focus on a specific instance, leading to comprehensive insights that a researcher would not find with a broader focus.
The case method can take many forms and combine various data collection methods. Such a range of data can also help a researcher understand the context of a specific event or how a similar individual or organization might respond to the same stimuli.
Case Study Example: A study on the impact of remote work on employee productivity and well-being in a tech company.
- Focus: The study focuses on a single tech company known for its rigorous adoption of remote work policies during and post the COVID-19 pandemic. This company was chosen because it represents a critical case in understanding the broader impact of remote work policies in the tech industry.
- Data collection: Structured and unstructured interviews of employees across different levels including management and staff. Non-participant observations of virtual meetings and remote work interactions. Review of internal documents such as emails, productivity reports, and employee satisfaction surveys.
- Analysis 1: Use data triangulation (i.e., compare interviews with observations and documents). Validate findings with participant feedback sessions.
- Analysis 2: Guided by theories of organizational behavior and concepts of motivation, job satisfaction etc. Understand the company's culture, its organizational structure etc. and how these factors interact with remote work dynamics. This includes analyzing the industry context.
- Report: Detailed description of remote working practices at the company and how remote working has affected employee well-being and productivity.
Ensuring Effortless Qualitative Data Analysis
With so many different forms of analysis to choose from, it’s vital that you have the right tools available to perform each one efficiently and reliably. Software like NVivo can help – offering accurate, efficient qualitative data analysis to help you search for meaning in your data.
Ready to enhance your research analysis? Speak with our experts to learn how you can use NVivo to dig deeper into your data.