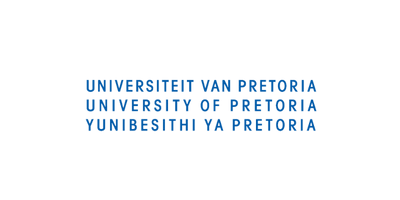
The University of Pretoria, South Africa, in collaboration with researchers from Utrecht University and the University of New Mexico, has used Lumivero's (previously Palisade) DecisionTools Suite software to create a low-cost and easily implementable model to estimate foodborne contaminations and human infections from the avian influenza H5N1 virus in Egypt and Nigeria. The output of this surveillance model, when combined with data generated by several international health organizations, will enable other African countries to better predict, monitor and intensify efforts to eradicate the spread of this highly contagious disease from animals to humans. This work was covered in the article, Development of Disease-specific, Context-specific Surveillance Models: Avian Influenza (H5N1)-related Risks and Behaviours in African Countries, published in April 2015.
Background
The avian influenza virus – or avian flu – is a fast-spreading infection that affects poultry and potentially people worldwide. While the virus has already adapted to other mammals, including ferrets, guinea pigs and cats, the risk to humans is still not completely understood. This makes monitoring and decreasing the rate of contact between infected poultry and humans critical – in particular, stopping exposure to the virus through the production and preparation processes of contaminated food. According to Dr. Folorunso Oludayo Fasina, a senior lecturer at the University of Pretoria’s Department of Production Animal Studies, it is critical to understand “how the virus gets into the food system, how it spreads and how it can be managed. To do this, we need risk assessment and exposure assessment, as well as a response model. Once we have this information, we can implement measures to stop the risks.”
As the University’s Department of Production Animal Studies has significant expertise in disease modelling and risk prediction as part of their epidemiological work, they allowed Dr. Fasina and his colleagues to create a model for foodborne contamination that was specific to Africa, where the virus has already infected 12 countries. The team studied both biological and cultural aspects, including food processing, trade and cooking-related practices, and collected data from more than 375 Egyptian and Nigerian sites including homes, local producers, live bird markets, village and commercial abattoirs and veterinary agencies. According to Dr. Fasina, “We took a ‘from the farm to the fork’ approach, and considered farms as well as livestock markets.”
Department of Production Animal Studies, University of Pretoria
Building the Model
“Risk mitigation and risk prediction remain some of the most useful tools with which to effectively control the continuous perpetuation of outbreaks in newer territories and eradicate it where it currently exists,” explained Dr. Fasina. However building this new model wasn’t an easy task, taking nearly two years to complete. Most of the existing information was qualitative, which made it difficult to set quantitative parameters, and the quantitative data they did find was inconsistent as it was often out of date, only available for other types of influenza or had been censored by the government. However after attending a training session for DecisionTools Suite in 2013, Dr. Fasina decided to use the software to generate the quantitative values they needed.
The team considered several factors with their model, from the concentration levels of the virus in infected meat and the likelihood of contamination between infected and non-infected meat, to differences between genders and age groups with regard to risk exposure. “We asked a lot of questions to generate this data,” explained Dr. Fasina. “This generated a significant amount of output, which required sensitivity analysis and some triangulation.” As a first step, the team used TopRank tool, part of the DecisionTools Suite, to analyze the sensitivity of each of the identified contributors to the overall risk. This helped the team understand which of the contributors were the most important.
Next, the team moved to the @RISK tool in the DecisionTools Suite to help predict the different ways the virus could be spread. Using Monte Carlo simulation, @RISK can quantify the probabilities of different outcomes – or infection rates – occurring, as well as determine the optimal preventive measures to mitigate the risk of animal-to-person infection. The team used six statistical probability distributions within @RISK to represent different inputs – or risk factors – for their model. They combined the simulated outputs from @RISK with statistical analysis to complete the model, using social data and outbreak information, including human demographic structures in Africa, socio-cultural and behavioral economics and knowledge, and attitude and perceptions or risks within those countries being investigated.
The results revealed numerous opportunities for the avian influenza virus to be spread, and found that the estimated risk for humans was higher than previously reported. “It is very easy for us to miss the influence of viral infections on a community, due to lack of awareness and under-reporting, so people may be more at risk than we’re aware of,” explained Dr. Fasina. “@RISK is a valuable tool to investigate these problems and do risk predictions either prospectively or retrospectively. Utilizing the outputs from models like this can help health policy planners and public health officials to take anticipatory measures to prevent future disasters associated with infectious diseases like the avian flu.”
Originally posted: Feb. 8, 2017
Updated: June 7, 2024