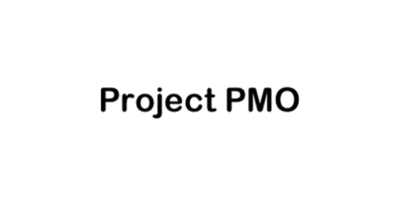
Meeting schedule milestones in a timely manner is paramount in any industry, but particularly important in those working with the Department of Defense, where deliverables can be a matter of national safety, and, where program delays can become very expensive due to large, highly compensated staffs. A major aerospace company was contracted by the Navy to build defensive missiles designed to ward off missile attacks from hostile nations such as North Korea, and was required to provide accurate estimations of their timeline and deliverables, including conceptual and detail designs, manufacturing, test, integration, and delivery.
To do this, the company previously used an out-dated schedule risk analysis method, and was informed by the Navy that they had to adopt a more quantifiably rigorous approach. To solve this dilemma, the firm brought on Jim Aksel from ProjectPMO of Anaheim, California, as a consultant to help adopt a more accurate method for evaluating their timeline.
“They didn’t have a Monte Carlo simulation tool,” says Aksel. “They were adding duration margins to many tasks without knowing if that amount of time was correct or to the correct tasks. It was simply a guesstimation.”
Lack of Critical Path in Aerospace Firm’s Missile Development Schedule
When Aksel first came on to the project, he and his team took a hard look at the company’s existing schedule for the missile development. “After examining the schedule, cutting up and taking out the ‘junk,’ we realized they didn’t have a credible critical path.” A critical path is the sequence of activities which result in the longest path through the project; this sequence is the key factor in determining how long the project will take. A delay to any task on the critical path extends the entire project duration. Aksel was able to separate the tasks in the timeline, remove unnecessary logic and date constraints, and determine a critical path, thus enabling the next step in the schedule risk analysis process—Monte Carlo simulation.
Determining Durations in a Project Schedule using @RISK’s Monte Carlo Simulation
Monte Carlo simulation performs risk analysis by building models of possible results by substituting a range of values—a probability distribution—for any factor that has inherent uncertainty. In the case of a schedule risk analysis, the variable containing uncertainty is the unfinished tasks’ remaining durations. Monte Carlo Simulation then calculates results over and over, each time using a different set of random values from the probability functions. Aksel chose Palisade’s @RISK software to conduct these calculations for the aerospace firm. Before these calculations could be done, Aksel needed inputs for the schedule model –specifically, a range of estimated durations of each task. To get the inputs, Aksel interviewed the engineers and other key players involved in the project for their best guesses on each task’s duration.
“We needed to give them some information about what exactly we needed,” says Aksel. To help them understand, Aksel used the analogy of your morning commute. “I want to know, on a typical day with typical traffic, what’s the earliest you’d get to work—not how long the drive would take on a Sunday morning at 4 AM,” he explains. Staying with the simile, he also wanted the latest time to work on a typical day – not the day when there is a truck turned over blocking the freeway. For the missile program, Aksel essentially wanted a range of what the typical duration would be, without the rare extremes. The @RISK software has the capability to process both types of distributions as part of its setup. Each task then contains three estimates of remaining duration: optimistic, most likely, and pessimistic. The values need not be symmetrical.
The aerospace engineers provided their educated estimations on each task in the critical path, and had quantifiable backup data (such as prior history of similar tasks or incremental bottoms up estimates). Aksel plugged these inputs into @RISK’s schedule model via Microsoft Project. Tasks not laying directly on a critical path were “banded” using percentages such as Most Likely Duration ±20% (again, the window does not have to be symmetrical). After running the simulation, they got a distribution of durations for tasks identified by @RISK, including estimated windows of dates for contractual program milestones such as System Requirements Review, Preliminary and Critical Design Reviews, and First Flight. From this information, the team was able to estimate (with numeric certainty) the probability of contractual milestones occurring no later than specific dates. These dates can then be used as a basis for performance incentive payments. This changed the timeline for deliverables.
Jim Aksel
Principal, ProjectPMO
Shorter, More Accurate Schedules for the Engineering Team
“Previously, the engineering team had just added 22 days of margin to a task to ensure enough buffer time to complete things,” reports Aksel. “But after running the Monte Carlo simulation, we found that 95% of the time, you would only need 10-14 days of margin time for that milestone.”
By cutting out the ‘junk’ tasks in the project schedule, and using @RISK to more accurately determine the durations between milestones, Aksel and his team were able to shorten some estimates by six to eight weeks!
Aksel says that the @RISK model outputs can sometimes surprise the experts. However, since the experts provided the data for the inputs, “you don’t squabble about the output” says Aksel. Instead “you get to process it.” He adds that one common question asked is, ‘Have we performed a sufficient number of iterations?’ Given the output of the model, and the desired level of precision, this becomes a simple mathematical calculation. “If there are not enough iterations, you can run the model again, or, determine the precision that exists in the current model and decide if it is sufficient,” he says.
Best Success: “True Belief in our Logic”
For this particular project, Aksel explains, the model matched fairly closely to the engineering team’s original estimates with only a few exceptions. “It helped solidify that we had true belief in our logic,” says Aksel. “That’s the best success we could ask for.” The program manager then has the unenviable task of making sure the team has the resources necessary to perform the tasks as scheduled without resource overloading—a.k.a., overburdening certain team members or groups. Clearing and avoiding resource overloads is a necessity in performing a credible analysis.
Aksel goes on to describe his favorite feature of @RISK: formulas, data, and models are all accessible and viewable in one place. “It lets me see what’s going on where,” he says. “I’m able to see everything in one place, so there’s less mystery. I showed the team the inputs and made sure there was consensus from everyone on all the inputs. It is important to include all stake holders in this process. When two stake holders, with different interests, can intelligently discuss the probability distributions, and the triple point estimates in play, then you know people are going to take the outputs of @RISK seriously.”
In conclusion, Aksel falls back on an axiom presented in his college statistics textbook: “You need to use statistics like a street light – for illumination – not like a drunkard who uses the streetlight for support.”