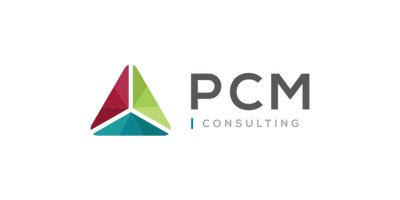
Assessing Financial Risk
Most studies on the economic viability of biogas plants only consider traditional financial indicators like Net Present Value (NPV) and Internal Rate of Return (IRR). "This puts investors at the risk of overlooking the volatility of a project, based on uncertain events," says Nagel. "Risk-based decision criteria can aid in identifying and mitigating the most influential risks." To do this, Nagel used @RISK’s Monte Carlo simulation software to better examine the effects of parametric uncertainty on the financial viability of biogas plants in her home country, South Africa. The simulation analyzed 8 biogas plant scenarios varying in energy output (measured in megawatts electric, or MWe) from 0.5 MWe to 6 MWe plant capacity. The biogas usage pathways considered include electricity generation through a Combined Heat and Power (CHP) unit and upgrading of biogas to bio- methane for application as vehicle fuel.
Model Inputs
Nagel gathered financial and technical data on functioning biogas plants via interviews and scientific journal articles to input into the Monte Carlo simulation. Next, Nagel calculated a baseline Discounted Cash Flow (DCF) analysis where she calculated the Net Present Value (NPV) of each project scenario.
Nagel next assigned probability distributions to the following key parameters:
- Project discount rate: reflects the rate at which capital can be made available for the project. South Africa’s current market rates vary between 7.18% and 9.68% for bonds of 10 years and longer. Therefore, a triangular distribution was used.
- Investment cost: these were based on a regression analysis to determine expected plant costs, with prediction intervals at a 95% confidence level.
- Annual revenue: determined by production rate and the product selling price.
- The production rate can vary because of un- planned maintenance or plant shut-downs, impurities in the feedstock, supply interruptions, etc. Nagel modelled this as a normal distribution with mean at the design rate and a 20% standard deviation, which is twice the design down-time.
- Nagel assumed the product selling price to increase annually in-line with inflation, at 5-6%.
The image shows the probability distributions used for key parameters in Nagel’s Monte Carlo simulation Minimize risk. Maximize potential.
Simulation Results
After running the simulations, Nagel found that at smaller plant capacities of 4 MWeq and below, the CHP plant scenarios had comparatively higher NPVs. However, larger plant capacities (5 MWeq and greater) the bio-methane plants have larger NPVs. 'At a capacity of 6 MWeq, both usage pathways had their greatest potential NPV, thanks to economies of scale," Nagel explains. “Bio-methane plants are more cost-sensitive, but also more profitable at larger plant capacities."
Figure 1: shows the probability distribution of NPV for bio-methane plants with capacities from 1 MWeq to 6 MWeq:
Figure 2 shows the probability distribution of NPV for CHP plants with capacities from 1 MWeq to 6 MWeq
In comparing the two probability distributions of the different plants, Nagel made the following observations:
"For a single income stream, the 6 MWeq plant scenario is the most attractive for both biogas usage pathways,” Nagel explains. “Although the probability for financial success is positive, it is less than an often-used level of 95%."
For both biogas usage scenarios, as the plant capacity increases, so does the probability of financial success and the value of the median NPV. "This is thanks to economies of scale,” Nagel explains. “As larger sales volumes will result in larger revenues while unit costs decrease."
However, uncertainties also increase at the larger capacities, with the normal distribution becoming broader at these places on the simulation. Nagel further evaluated this by looking at parameter dis- tributions with the greatest effect on project NPV:
Brigette Nagel
Parameters with Greatest Influence on NPV:
"From these graphs, you can see the financial risk for smaller plants is dominated more by investment costs, while the financial risk for larger plants is dominated by revenue-linked parameters," says Nagel.
To further understand the effects of variations in the most influential parameters, Nagel focused on annual revenue, annual fixed cost, and investment cost, while keeping all other parameters constant:
Annual Revenue:
Nagel found that, as the variance increases, the bell curve broadens out
Annual fixed cost and investment costs: @RISK
Nagel also showed that how investment costs and annual fixed costs varied with increasing plant capacity:
"I found that, as plant capacity increases, the range of possible cost values also increases,” says Nagel. “This is linked to limited data availability at larger plant scales, as most existing plants are small scale. These two para- meters therefore contribute to increased uncertainty on actual plant cost at larger plant capacities."
Take-home Findings
After examining @RISK’s simulations, Nagel arrived at key insights for the South African biogas industry. "Many other industries require a 95% probability of financial success. If that is the benchmark, none of the plants evaluated would merit investment," says Nagel.
However, plants could take steps to encourage invest- ment. For smaller plants, Nagel says, they must work on minimizing risks associated with investment costs and operational costs. "For large scale plants, the greatest focus should be on maximizing the value of the energy product, such as finding a client that would pay a premium for green energy."
Benefits of @RISK
For Nagel, this post-graduate work would not have been possible without @RISK’s robust simulations and user- friendly interface. "This software allowed me to explore all the variables and drill down into the key reasons biogas plants might or might not succeed," Nagel says. "I will continue to rely on this system for future analyses that require both rigorous number crunching and elegant visualizations."