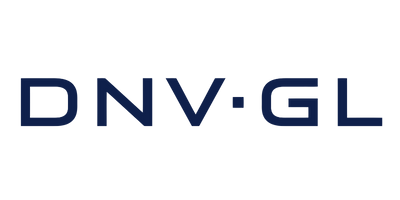
Det Norske Veritas (DNV) is a global provider of services for managing risk for a variety of industries worldwide. The organization was interested in comparing and contrasting risk factors and ROI of non-traditional and traditional energy sources, and utilized @RISK to establish a baseline of probabilities for a number of risk factors associated with each system.
About DNV
Established in 1864, DNV primarily provides technical assistance to ship owners, builders and operators to enable them to comply with certifications and classification services required to meet the technical regulations for the shipping industry. DNV also provides third party consulting services to companies in the energy industry to help them build offshore structures such as wind turbines.
Technological, Regulatory Shifts Require Constant Attention
DNV clients are subject to elements of change, which are often outside of the clients’ control. Whether adapting to technical transitions in the maritime and oil industries, or considering the regulatory adjustments experienced in the healthcare and food and beverage industries, DNV must determine the potential risk factors created when the status quo is altered. Risk factors can change quickly, which requires DNV to adjust its projections accordingly.
Clients trust DNV to offer accurate forecasts of risk, and DNV meets this challenge through the utilization of Monte Carlo simulation with Palisade’s @RISK software. @RISK works by providing a range of probability distributions to represent uncertain variables, and then computing hundreds or thousands of different scenarios. A probability distribution is simply a range of values with greater probability of certain values occurring than others. The normal distribution, or “bell curve,” is a common example. The values around the center – or mean – of the distribution are more likely to occur than the values at the ends, or tails.
DNV Utilizes @RISK to Examine Risk in Non-Traditional and Traditional Energy Systems
With any energy system, inherent risks can be found, and those risks can create instant emergencies and long-term drawbacks. DNV set out to quantify risk of non-traditional energy systems (wind and solar) and traditional energy systems (nuclear waste storage, oil and gas, nuclear and CO2 recycling). For this project, the organization utilized @RISK’s triangular distribution in its projection models. The research involved a three-step process:
1. Identifying model inputs. These inputs are uncertain factors and vary by model. In this case, inputs such as weather variables, energy costs, production rates, equipment deterioration and location of energy source extraction were considered. 2. Creating a simple economic projection to determine if the model is fundamentally accurate. 3. Conducting a sensitivity analysis to determine the most important factors driving risk in the model.
This process was repeated continuously to refine each model. Once a reliable model was established, DNV inserted probabilistic variables to produce more detailed statistical analyses and identify outcome probabilities of each energy source.
Davion Hill
Senior Researcher, DNV
What @RISK Helped DNV to Determine
With the utilization of @RISK, DNV found useful information regarding various risk factors in non-traditional energy sources.
Wind and Solar The region in which the equipment — wind turbines and solar voltaic panels — was built had a strong effect on its net return on carbon investment. For example, solar panels made in a coal intensive electric grid would be challenged to pay back that carbon if they weren’t deployed in a sunny area.
Nuclear Waste Storage The analysis was simplistic, but nuclear waste storage tanks saw sensitivity factors flip depending on whether they were examined the liquid phase or vapor phase.
Oil and Gas For the offshore riser calculation, the probabilistic analysis helped DNV to determine which flow conditions were most severe in terms of corrosion rate.
CO2 Recycling Probabilistic analysis led DNV to change its cell design. They began with a very consumables-intensive process which consumed a lot of sodium hydroxide (NaOH). The value chain model showed that NaOH consumption was a major negative sensitivity factor in the net present value projection for the system. “This helped us change to a less NaOH-intensive electrochemical process,” said Davion Hill, senior researcher at DNV. “We are now using the sensitivity analysis to identify alternatives that will help us bring down the energy costs. This iterative analysis has been very powerful in the shaping of this project.”
@RISK Simplifies Explanation of Risk
Gathering of risk information is only as useful as the ability to translate and explain it to those who require the data. DNV found one of @RISK’s strongest attributes to be the straightforward nature in which the analysis is presented.
“Risk is part of everything we do. We are very committed to our role as an objective third party and we must enable trust in the relationships between our customers. Therefore, when we present risk to our customers, we prefer to do it in a way that is tangible and relevant to the context of the situation. Using tools that quantify risk is an important part of that service,” said Hill. “Groups that are typically running process models or economic projections value the sensitivity analysis capability of @RISK.”
For DNV, @RISK assisted the organization to recognize variables that impact the outcome of the energy systems identified earlier. “This helps us understand the process better, and it also helps us communicate with the customer intelligently,” said Hill. “With continual refining and improvements, we identify improvement areas while simultaneously improving the quality of our own work.”