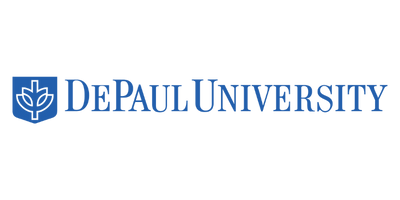
With more than a trillion dollars risked worldwide on sports gambling every year, there certainly is interest—on behalf of bettors, at least—to turn the odds away from bookmakers. DePaul University professor Clayton Graham drafted and entered a research submission entitled, “Diamonds on the Line: Profits through Investment Gaming,” for the Ninth Annual MIT Sloan Sports Analytics Conference in February 2015. The prize winning paper, which topped the “Business of Sports” track and was in the elite “Final Four,” utilized Palisade’s DecisionTools Suite in determining critical probabilities to create such a model for baseball wagering.
Clayton Graham is an Adjunct Professor at DePaul University’s Driehaus College of Business and a Management Consultant with Advanced Analytics LLC. His professional focus is applying statistics and mathematical modelling to analyze, evaluate and develop operational strategies and tactics. Clients include school districts, universities, major corporations, sports entities, and governmental agencies.
The MIT Sloan Sports Analytics Conference provides an annual forum for over 3,000 executives, leading researchers, and students to discuss the increasing role of analytics in the global sports industry. Graham’s research sought to calculate a team’s probability of winning an individual baseball game, and the economic consequences of each wager based upon the game’s betting line. Additionally, he attempted to determine the optimal bet size, subject to the risk tolerances of the investor.
The research required five critical steps:
- Building a production function that generated the scoring characteristics of each team, and, in turn, the resultant probability of winning
- Incorporating the market betting lines (Money Line) of each game resulting in the determination of payoff or loss
- Establishing an interactive economic relationship between the production function and betting line
- Creating a risk/return-based investment function compatible with the model
- Quantifying the results
Building the Predictive Production Function
This initial step required a pragmatic selection of input and output variables. First, Graham considered a very fundamental question: What is the batter’s purpose? Simply, it is to get on base and drive other runners around to score. Those inputs include the proportional measures of singles, doubles, triples, home runs and bases-on-balls. While there are other inputs such as hit-by-pitch, dropped third strike by catcher, etc., Graham found those situations to be of little significance in the prediction mode. At the heart of the model is the history of each batter/pitcher match up.
It is worth noting that Graham wanted a more accurate representation of scoring beyond runs per game, and focused, instead, on runs per out. His reasoning was that not all baseball games have the same number of innings (outs). If the home team is ahead after the visiting team completes their half of the ninth inning, the game is over after completing just 24 outs, as opposed to a full game consisting of 27. Should a game go into extra innings, the number of outs will exceed 27.
Once the runs per out were determined, Graham then applied a “park factor” adjustment, which measured the difference between runs scored in a team’s home park and road games. To equalize the influence of the park factors between competing teams, expected value of run production needed to be scaled upward or downward. Since the starting pitcher and batters likely played in all fields previously, the park factor was a scaled convex combination of the two parks’ factors.
Graham also determined that the average runs per out during the last three innings of a typical game equalled 91 percent of the runs tallied in the first six innings. Once he had the ability to calculate the potential run output, he could apply that data to the betting line of each game.
AMSAA Mathematician and Statistician
Defining the Betting Line
Popular American sports, such as basketball and football, utilize a point spread that determines how many points one team is favored over the other in a given contest. For example, if the Boston Celtics are 3-point favorites over the New York Knicks, a bettor wishing to place a wager on the Celtics would need the team to win by at least four points to win the bet. If the Celtics win by three points, the bettor’s initial wager is returned and if they win by less than three points or lose the game, the bettor loses the wager.
However, baseball’s “Money Line” is different than the “Spread” commonly used in basketball and football. Consider the following betting line if the Detroit Tigers hosted the Seattle Mariners:
*Seattle -113 *Detroit 105
In this scenario, Seattle, by virtue of having the lower, value is the favored team. The bettor would risk $113 to win $100. In contrast, betting $100 on Detroit would return $105.
Examining Economic Relationship: Production Function and Betting Line
Using formulas based on the batter-pitcher matchups, Graham determined the probability that either team would win the game. Then, Graham applied those probability functions to economic outcomes of a sports gaming investment. Two measures were derived; the first is the standard expected value of return on investment (EVROI), which yields either a positive or negative number. The second is the “edge”, which is simply the difference between the probability of winning and the implied probability of winning from the betting line. With these, one has the two principal elements for investment: Probability of winning and competitive edge.
Creating Risk-Return Investment Function
Next, Graham utilized the probability of winning and the edge to derive the level of investment (percent of Bankroll) subject to investor-imposed risk tolerances. This significantly limited the number of games worthy of investment.
Quantifying the Results
Once the model was complete, an initial bankroll of $1,000 was used to place wagers (about two per day) on Major League Baseball games, beginning on June 16, 2014 and through the conclusion of the World Series on October 29, 2014. Key results included:
*Only 23 percent of MLB games warranted a wager, based on probability of winning and the edge. *Games with a favorable determination won 68 percent of the time. *Wagers resulted in a 35 percent return on daily capital put at risk (bet). *The initial $1,000 investment grew by more than 1,400 percent during the season (a profit of $14,252).
“Palisade’s DecisionTools Suite was invaluable to the success of this exciting project, as it quickly and easily computed the myriad statistical scenarios,” said Graham. “Baseball has a seemingly infinite set of possibilities with each at-bat, and the intricacies of determining what may happen would be impossible to determine manually, with any degree of expediency. DecisionTools Suite is also very easy to use and intuitive because it operates in Microsoft Excel. I can say, without hesitation, that this project would not have been possible without DecisionTools Suite and the technical support Palisade offers.”