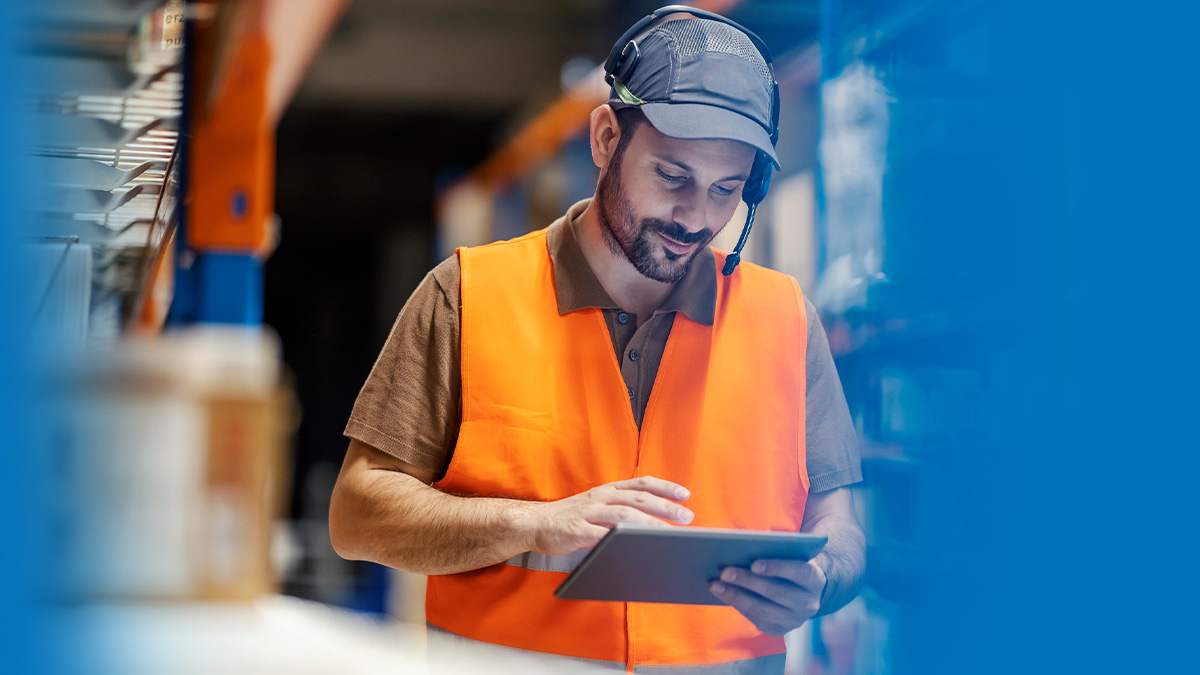
How to Optimize Production and Inventory and Improve Tolerance Stacking Through Risk Analysis and Forecasting
Supply chain management issues continue to be top of mind for manufacturers, specifically issues relating to optimizing production and inventory. According to a survey of more than 200 manufacturers published by Deloitte in September 2022, part shortages impacted 57% of manufacturers during the 18 months prior to the survey, while 31% of manufacturers cited “excess inventory due to poor forecasting” as a key operational concern in the near future.
In our first article looking at the supply chain applications of Monte Carlo simulation in supply chain management for manufacturing companies, we focused on how they can help with risk analysis and decision-making during uncertain times. In this article, we’ll look at how two manufacturing firms have used probabilistic analyses with Monte Carlo simulation to optimize their material stocks and engineering processes – leading to more efficient operations, improved cost control, and better communication with vendors.
Monte Carlo Simulation: A Quick Refresher
Monte Carlo simulation is a mathematical analysis technique that dates back to the Manhattan Project. When coupled with modern computing power, Monte Carlo simulation creates models that forecast the most likely outcome of a situation given certain conditions. Crucially, these simulations also account for random chance, which is why the technique is named after the casino in the Principality of Monaco.
This technique is used in weather forecasting, physics, telecommunications, financial investing, insurance, and many other industries to drive optimal decision-making and clarify risks. For manufacturers, risk analysis tools that leverage Monte Carlo simulation, like @RISK and Evolver, can also help drive supply chain management efficiencies.
Mettler Consulting: Optimizing Production and Inventory Through Improved Forecasting
Don Mettler is an enterprise resource planning (ERP) consultant that specializes in working with large manufacturers who want to make more efficient use of resources. One of Mettler’s clients, a corrugated cardboard manufacturer, needed a way to better inform production on a line that produced boxes for shipping fresh produce. Unpredictable demand for the produce box frequently led to overproduction, leaving the client with significant excess inventory. Mettler set out to help this client balance fluctuating demand with fixed plant capacity.
Using @RISK and Evolver, Mettler was able to develop a production schedule optimization model that drew on numerous inputs including daily order data from the client’s ERP software.
“Every manufacturing company should use schedule optimization,” Mettler told Lumivero. “You have a finite set of resources, and you want to maximize gain while minimizing the cost of handling those resources. It’s a problem just made for… Evolver.”
The model Mettler created ran analyses using Monte Carlo simulation based on the last 52 weeks of data. By updating the model every night, Mettler’s client can better anticipate demand –adjusting production only to the number of boxes it will need to ship that coming week.
Pre-Formed Line Products: Better Tolerance Stacking Through Monte Carlo Simulation
Pre-Formed Line Products (PLP) is an Australian manufacturer of infrastructure equipment for the energy and telecommunication industries. Their products are used to connect and secure power and communication lines in over 20 countries, and they rely on external suppliers for components that make up many of those products. When the PLP engineering team needs to combine parts from more than one supplier, they conduct tolerance stacking to determine to what extent each component can deviate from their requirements and still be usable in PLP’s final product.
For example, one of the products PLP creates is called a “spreader rod” which holds low-voltage power lines in place during windy conditions. Two components from suppliers are used in the spreader rod —a fiber-reinforced plastic rod made through pultrusion and a metal spring. For the spreader rod to function, the diameter of the plastic rod component must be smaller than that of the metal spring.
Traditionally, PLP’s team would perform tolerance stacking by separately calculating the maximum deviation for the diameter of the rod and the maximum deviation for the size of the spring. Then they would split the difference and tell each supplier they had to meet the same standards. However, given the different manufacturing processes involved in making a pultruded plastic rod and a metal spring, PLP’s team realized that this approach could place significant burdens on one supplier compared to the other. Additionally, PLP frequently found itself rejecting parts because a supplier could not meet the standards set by this type of tolerance stacking.
That’s where Monte Carlo simulation came in handy. PLP’s engineering department conducted their own random-sample measurements of rod and spring components also in addition to requesting some statistical process control data from their external suppliers. They used this data to develop a probabilistic model using @RISK and its GoalSeek tool.
By running multiple simulations, the PLP team was able to better define specific tolerances for each external supplier — tolerances that better accounted for their individual manufacturing processes. The result was fewer rejected components and better communication with the suppliers.
Optimizing Production and Inventory with Monte Carlo Simulation
Probabilistic analysis tools like @RISK, Evolver, and GoalSeek can provide manufacturers with solutions for optimizing production by improving day-to-day operations and external supplier relationships. Find out more about how your organization can benefit by downloading our free example models for different scenarios today: