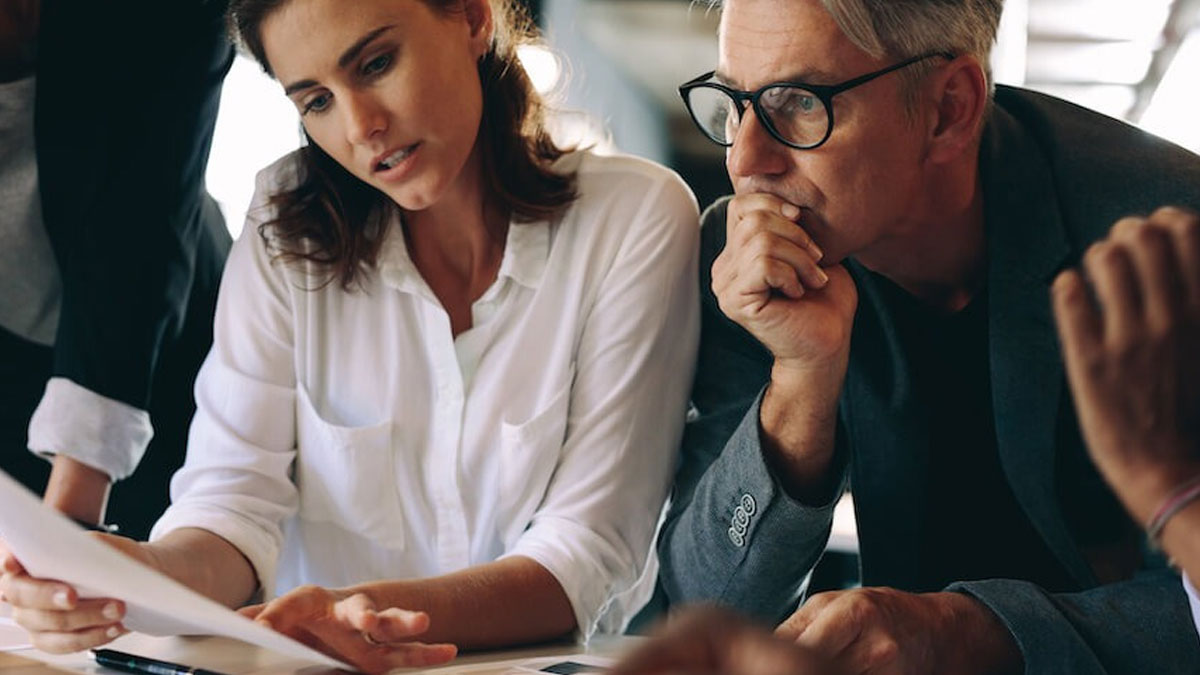
Organizations of all types use analytics to approach decision-making more objectively, accurately, and confidently. These techniques help decision-makers at all levels to be better-informed and to think in unbiased terms. Study after study shows that businesses who apply some level of rigor and analysis to complex decisions routinely enjoy better outcomes.
But what is analytics? To some, it means data analysis, plain and simple, and is basically a fancy synonym for statistics. However, the concept has been broadening in recent years to encompass a wide range of mathematically-based approaches to decision support.
Types of Analytics
There are now four generally recognized types of analytics which form a common language among not only operations researchers, but also among the current generation of decision-makers in business, healthcare, engineering, and government. These types are descriptive analytics, diagnostic analytics, predictive analytics, and prescriptive analytics. The order in which you consider them matters, as each represents a stage in a progression from simply describing something that happened, to predicting and eventually attempting to control what will happen in the future. As such, this “Analytics Pyramid” represents a path from hindsight to foresight – and it’s this foresight which will set apart tomorrow’s success stories from its failures.
Descriptive Analytics
What Happened?
At the base of the Analytics Pyramid, descriptive analytics answers the question “What happened?” It is the collection, organization, analysis, and presentation of historical data in a way that is easily understood. Descriptive analytics is focused only on what has already happened in a business or organization and, unlike other methods of analysis, it is not used to draw inferences or predictions. Rather, descriptive analytics forms a starting point to prepare data for further analysis.
Generally, descriptive analytics uses simple statistical tools (such as mean, percent changes, and standard deviation) from statistical analysis rather than complex calculations. Line graphs, pie charts, and bar charts are used to present findings for consumption by a wide business audience. Summarizing sales, operations data, or economic trends are examples of applications of descriptive analytics.
Diagnostic Analytics
Why Did It Happen?
Diagnostic analytics answers the question “Why did it happen?” It’s a more advanced form of analytics characterized by drill down, data discovery, data mining, and correlation techniques. It’s the next logical step once you understand what happened. As in descriptive analytics, diagnostic analytics lends itself to graphical analysis for communication with others. Applications of diagnostic analysis include determining which market segments or products contributed most to sales, which factors led to operational results, and what individual sectors of the economy were doing to drive overall economic performance.
Specific diagnostic methods include sensitivity and scenario analysis, as well as statistical inference. Sensitivity analysis lets you see how any given individual variable impacts the outcome of interest, while scenario analysis considers combinations of independent variables working in tandem. Statistical inference applies algorithms to fill in gaps of understanding in a data set.
Predictive Analytics
What Will Happen?
Predictive analytics answers the question “What will happen?” and shifts focus to predicting and understanding what could happen in the future. One way to do this is by analyzing past data patterns and trends by applying statistical techniques such as regression and forecasting procedures. Another way to utilize data for predictive purposes is through neural networks, a form of machine learning which examines historical data sets to develop models capable of make predictions from new, incomplete information.
Predictive analytics do not strictly require data, however. Monte Carlo simulation, for instance, can be effectively used to calculate the probability of different outcomes occurring, even if models are constructed in the absence of data. This is because Monte Carlo is able to utilize theoretical probability distributions, which can be defined with either data or with expert opinion alone. The simulation itself then generates data which is used for predictive analysis.
This methodology empowers executives and managers to take a more proactive, data-driven approach to business strategy and decision making. Businesses can use predictive analytics for anything from forecasting customer behavior and purchasing patterns to identifying sales trends. Predictions can also help forecast such things as credit fraud, supply chain bottlenecks, and inventory demands.
Prescriptive Analytics
How Can We Make It Happen?
At the peak of the Analytics Pyramid, prescriptive analytics answers the question “How can we make it happen?” or “What should we do?” This is the final logical step in the progression to foresight, and is the one that calls organizations to action, helping executives, managers and operational employees make the best possible decisions based on the data available to them. It goes a step further by recommending the best possible courses of action.
Prescriptive analytics leverages a number of techniques and tools such as optimization, Monte Carlo simulation, decision trees, AI, and machine learning algorithms. These tools can anticipate what, when and, importantly, why something might happen. After considering the possible implications of each decision option, recommendations can then be made as to which decisions will best take advantage of future opportunities or avoid risks. Essentially, prescriptive analytics predicts multiple futures and, in doing so, makes it possible to consider these outcomes before any decisions are made.
Optimization and Monte Carlo simulation are key examples of prescriptive analytics. Resource allocation applications are common throughout science and industry and involve deciding how – and when – to distribute limited resources such as capital, time, and people. There is no clear answer in these cases, and data analysis alone won’t provide the solution. Optimization algorithms, often using artificial intelligence, will try and adapt different allocation solutions to arrive at a recommended strategy. What’s more, Monte Carlo simulation can be used to enhance the recommendation by providing insights into the probabilities of different outcomes occurring for each solution set. Finally, optimization can be paired with neural network prediction to set probabilistic constraints on the solution sets attempted.
Decision trees are another example of a prescriptive process. By allowing decision-makers to map out multi-stage decisions and apply probabilities to different outcomes (often derived from earlier statistical analysis), tree models can calculate expected values and make recommendations at each decision point in the process. As with optimization, they can be further combined with Monte Carlo simulation to more accurately depict what are in reality unlimited possible futures as opposed to a finite few end points.
The Analytics Pyramid and Data
The Analytics Pyramid summarizes this analytics progression. While it is common to start from a place of analyzing existing data, it is not necessary or always possible. It’s important to realize that analytics does not require data, and that a lack of data should not stop anyone from applying analytical rigor to a decision-making process. Indeed, organizations perform predictive and prescriptive analyses every day without data – the very nature of business problems often requires making decisions in the face of the unknown. Innovation demands risk-taking, and risk-taking usually means there is no data because nobody has done it before.
The Analytics Pyramid, showing the four types of analytics from hindsight to foresight.
Summary of the Types of Analytics
Democratization of Analytics
While descriptive and diagnostic analytics have been used for decades, predictive and prescriptive forms are still newer concepts in many industry sectors and geographic regions. Although some predictive and prescriptive methodologies may have been invented after the core statistical methods on which they are based, they have gained rapidly in popularity in recent decades and are widely available at the desktop level for the everyday user. Advances in computational power and software technologies mean that decision-makers at any level can now apply any of these analytical stages directly in their spreadsheet models.
Analytics with Lumivero
Lumivero’s DecisionTools Suite and XLSTAT software puts powerful analytics at the fingertips of any Excel user. The Suite and XLSTAT offer tools for all types of analytics methodologies, from the descriptive to the prescriptive. Explore the tools for yourself by requesting a free demo of DecisionTools Suite and 14-day trial of XLSTAT.