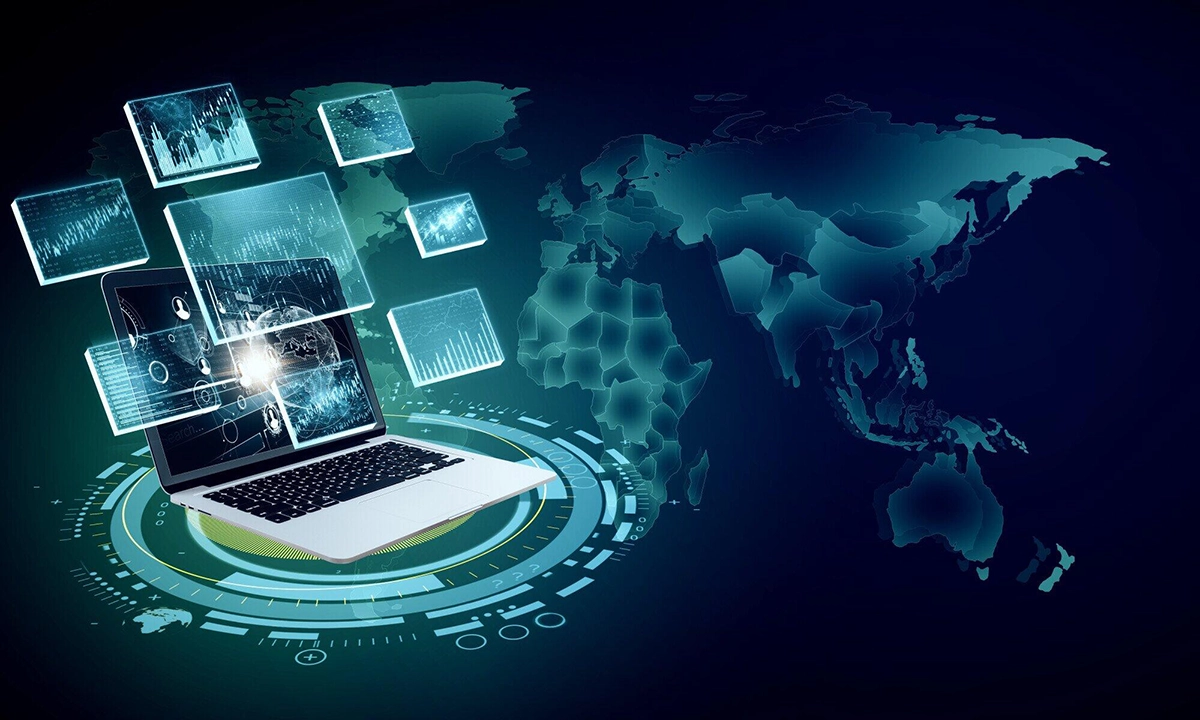
With part shortages causing issues at more than 50% of all manufacturers, it’s no surprise that they are looking for better ways to predict where supply issues might crop up. Even the top manufacturing companies need better, data-driven techniques to help them stay ahead.
Are you using all the tools at your disposal to optimize production and improve your manufacturing supply chain?
The article below explains how applying Monte Carlo simulation in manufacturing contexts can help you see all possible scenarios which help develop process improvements – saving time, money, and stress. Read on to learn the role of predictive manufacturing analysis and what it can do for you.
The Role of Monte Carlo Simulation in Manufacturing
Monte Carlo simulation (MCS) is a method of predicting the most likely outcomes from running thousands of possible scenarios with random variables. Such simulations use a series of probability percentages to work out how likely different outcomes are to occur. An analyst can run thousands of these simulations and derive conclusions about the future of their business.
The Monte Carlo method is not limited to assisting in finance, construction, and energy. Other areas, such as the manufacturing sector, can also benefit from its use. It acts as a critical tool for risk management and decision-making, helping people mitigate said risks that might have otherwise gone unforeseen.
Running these simulations results in more than a single outcome. The purpose of the Monte Carlo technique is to allow people to see probabilities of all possible outcomes coming to pass.
This is beneficial in a range of industries, especially in manufacturing where a single decision can be the difference between making a profit or taking a loss. Monte Carlo simulation is often used in manufacturing for supply chain and logistics optimization, forecasting, and pinpointing risks.
Read Monte Carlo Simulation 101 >>
What the Monte Carlo Method Offers Manufacturers
With access to a Monte Carlo simulation's results, risk managers can:
- Plan for the most likely adverse outcomes to mitigate risk to the business
- Adjust plans based on emerging knowledge to improve strategic planning
- Adjust simulations in real-time to get the best predictions possible
- Analyze uncertain variables to create primary and backup plans for strategic planning
- Communicate the range of possibilities to shareholders
Studies such as those by Farooq, S., Naseem, A., Ahmad, Y., et al. (2024) have shown that Monte Carlo simulation allows for "improved strategy for prioritizing risks." In their study, MCS discovered five times as many high-risk outcomes as conventional methods.
Such vital information can significantly help a company that wants to ensure its financial safety. For example, the results could inform a firm that while they’re likely to meet all production targets this quarter, they should also consider diversifying their strategies to brace for less optimistic performances in more severe recessions. Conclusions such as these mean businesses can take confident steps forward toward protecting their future.
Setting Up Your Monte Carlo Simulation
When you plan to run Monte Carlo simulations, you should perform the following steps:
- Identify the variables and how likely they are to occur as a set of probabilities
- Use powerful risk analysis tools like @RISK from Lumivero to define the simulation inputs
- Refine the model parameters to ensure they mirror real-world conditions Run as many simulations as is cost-effective to provide a range of results
- Analyze the simulation results and use them to make data-driven decisions
It is also imperative to make sure that the setup process uses accurate information to ensure the best results.
Identifying Crucial Variables for the Simulation
Begin by determining the factors that could impact your goal. Examples include:
- Machine efficiency
- Production capacity
- Raw material availability
- Worker productivity at different times of the year
You also want to consider the economic conditions of the business and worldwide. Consider:
- Budget limits
- Market demand for your product
- Price volatility
- Supply chain disruptions
- Exchange rate fluctuations
For example, consider a manufacturing plant producing a new type of computer chip in an unstable economic climate. You might input factors such as machine efficiency and expected processing times, raw material availability, and worker productivity, to name just a few – each subject to its own set of uncertainties.
Monte Carlo doesn't provide a definitive answer but a vivid tapestry of likely scenarios by running multiple simulations and offering possible outcomes – allowing you to make an informed decision.
Defining and Setting Input Distributions
With the Monte Carlo method, input distributions are used instead of input variables to better model how inputs with significant uncertainty could behave and influence the simulation outcome. This method ensures the outcomes are more likely to predict what might occur in the real world.
You can also use historical data to ensure the most accurate simulation possible. This is not to say the future will proceed precisely as the past has, but it may help describe predictable patterns in your business.
You will also need to:
- Choose the most suitable probability distribution for each input
- Calibrate any additional parameters
- Communicate with industry experts to ensure the distributions are accurate
Taking these steps will give you more accurate results and can help you make better predictions.
With software such as @RISK, you can define these inputs, set your model's parameters, and watch as @RISK’s distribution graphs display the range of possibilities.
Optimizing Novelis R&D Projects
For example, Novelis, the world's largest aluminum-rolling and recycling company, relies on Monte Carlo simulation to help rank its R&D projects by risk.
Novelis recognized the need for an improved structure around the risk evaluation of projects. As such, they could not communicate which areas of the company presented the highest risk – vital information for all key stakeholders. The lack of information presented a significant danger to the potential ROI of each project and, in many cases, could have even led to major losses.
By using @RISK to analyze how each link in their production chain influenced one another, they could collect data on the risks present across all work within their company. They then iterated on processes and allocated resources to ensure manufacturing efficiency improvement.
Confidently Implement Advanced Simulation Techniques in Manufacturing
Monte Carlo simulations can help you better manage risks and uncertainties in your business. The conclusions you draw from them can lead to better data-driven decision-making and an increased ability to overcome even significant challenges.
Lumivero offers user-friendly and efficient methods for running a Monte Carlo simulation in manufacturing with our @RISK and DecisionTools Suite analysis software. These tools allow you to run simulations on your datasets all within Microsoft Excel.
As you approach new manufacturing hurdles, consider the ways in which this timeless methodology, when coupled with the world’s leading risk analysis software @RISK and DecisionTools Suite, could revolutionize your approach to decision-making.
Request a demo to learn more about how you can revolutionize your manufacturing process and help you prepare for the future, today.