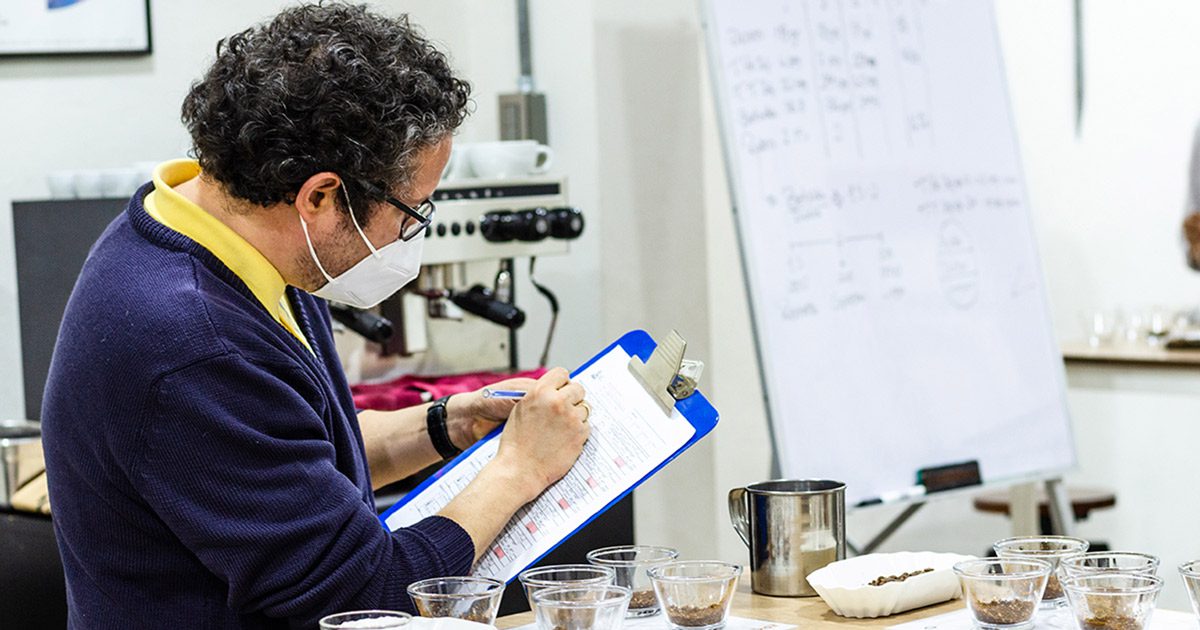
When you’re launching a new food product, sensory experiment feedback from consumers is key. However, sensory experience of food – different nuances of texture, flavor, and smell – can be highly subjective and varied among individuals. How can researchers group their consumers using sensory clustering methods?
Dr. Fabien Llobell, Lumivero’s Head of Quantitative Research, has made sensory data analysis a focus of his research. In his webinar, “Analyze Consumer Data with XLSTAT,” presented at the Lumivero Virtual Conference, Dr. Llobell shared an innovative analysis technique using XLSTAT that solves this problem by using RATA (or “Rate-All-That-Apply") data analysis method for survey data generated during sensory experiments for a raspberry coulis.
This article explores how Dr. Llobell’s development in XLSTAT made this breakthrough in sensory data analysis possible.
Continue reading or watch the webinar on-demand to learn more.
What is RATA data analysis?
RATA data analysis is a survey or sensory evaluation technique that allows the graded assessment of multiple attributes of a product. Assessors – in this case, tasters trying five different raspberry coulis sauces – were presented with a survey listing various qualities for each product. Each attribute had a rating scale associated with it, and assessors could check off any attribute that applied to the product they tried—then rate its intensity on a scale from zero to 3.
RATA analysis builds on a similar methodology, CATA (or “Check-All-That-Apply”). RATA offers deep insight because it allows researchers to capture data on how intense a characteristic is as well as whether it’s present. RATA analysis can also be less burdensome on assessors than surveys where they must rate every attribute – they can skip any attribute they think the product doesn’t have.
However, RATA’s detailed data output makes analyzing surveys based on it more complicated, especially when it comes to grouping assessors into clusters of similar sensibilities.
Analyzing sensory data and comparing responses between individual assessors
Dr. Llobell’s case study had 97 assessors give responses and ratings about the raspberry coulis sauces they tried. Each assessor’s set of data is known as a block. To define the similarity between assessors, he applied the Salton-Caussin formula to determine to what degree two assessors responded in the same way.
Running this analysis across all assessors allowed Dr. Llobell to generate a similarity matrix showing how well each assessor agreed with any of the others in the survey group. From there, it was a matter of defining clusters.
Building out clusters and sensory maps
Dr. Llobell had already invented CLUSCATA, available in XLSTAT, as a popular method for clustering respondents based on data from CATA surveys. But how could he account for the additional complexity and nuance provided by the RATA survey method?
To solve this challenge, he developed a partitioning algorithm which sorts assessors into clusters based on the homogeneity of their answers across all attributes – and this method is now coded in XLSTAT inside RATA data analysis.
RATA cluster analysis not only allows researchers to develop consumer clusters from this type of sensory data. It also allows them to develop sensory maps for each product based on assessors’ responses. These maps visualize the links between each product and the attributes mentioned in the survey data, as shown in this example for raspberry coulis:

Make your own data breakthroughs with XLSTAT
Interested in learning more about how you can use XLSTAT for more effective analysis of sensory data – including RATA survey responses? Request a demo of XLSTAT today.